Generative AI in Finance: Automating Reports, Fraud Detection, and More
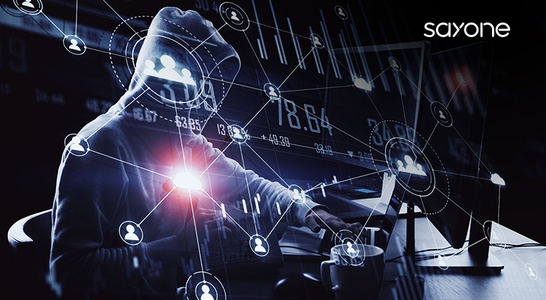
Share This Article
Table of Contents

Subscribe to Our Blog
We're committed to your privacy. SayOne uses the information you provide to us to contact you about our relevant content, products, and services. check out our privacy policy.
Struggling to keep up with manual processes while fraud risks climb?
You’re not alone.
Over 86% of banks are already implementing generative AI solutions, and 60% of finance teams plan to adopt it by the financial year end of 2025
With customer expectations for hyper-personalized services rising, static workflows simply can’t compete.
How does generative AI in finance work?
Outdated methods drain resources and leave gaps in compliance. But generative AI changes the game, automating reports in seconds, spotting fraud patterns humans miss, and scaling customer insights without hiring armies of analysts.
Why settle for reactive solutions when AI can predict risks before they escalate?
This isn’t hypothetical. By 2033, The annual growth rate of Generative Ai in the banking sector is predicted to reach 32.8% and 70% of customer experience teams will integrate generative AI to boost engagement.
Your competitors aren’t waiting and neither should you!
Let’s break down how this technology transforms finance operations, starting with the four areas where it’s rewriting the rules today.
How is Generative AI used in finance? Best Use Cases
Generative AI enables small and mid-sized financial institutions to compete with larger players through cost-effective automation, personalized services, and scalable solutions.
Below are practical use cases tailored for community banks, credit unions, and regional lenders:
1. Loan Processing & Underwriting
You can create automated loan processing applications using Generative AI to guide customers through loan applications, verifying income and credit data via natural language conversations.
Generative AI is streamlining loan applications and underwriting, leading to faster approvals and improved risk assessment:
- Guided Application Process: AI chatbots assist customers through the loan application, answering questions and helping fill out forms in real-time.
- Natural Language Processing: AI systems can conduct natural language conversations to verify customer information and identity, making the process more user-friendly.
- Automated Credit Memos: Generative AI can auto-generate sections of credit memos, including executive summaries, business descriptions, and high-level financial analyses.
- Risk Profile Analysis: AI algorithms analyze vast amounts of data to assess applicant risk profiles, considering traditional and alternative data sources.
- Approval Time Reduction: One of our fintech clients reduced loan approval times by 50% using AI to generate credit memos and analyze risk profiles.
- Risk Assessment: AI evaluates alternative data (e.g., cash flow patterns, utility payments) to expand credit access for thin-file borrowers, minimizing defaults.
2. Hyper-Personalized Customer Engagement
24/7 Virtual Assistants and AI-driven chatbots handle routine inquiries (e.g., balance checks, payment reminders) while flagging upsell opportunities.
Financial institutions can deploy a multilingual chatbot, cutting call center volume by 35% and boosting cross-sell rates.
Here are Use Cases from top banking institutions
- Bank of America's Erica chatbot processes 56 million monthly engagements, proactively suggesting budgeting tools when users exceed spending limits.
- Capital One's Eno reduces call center volume by 40% through instant resolution of balance inquiries and payment issues.
- Raiffeisen Bank's multilingual chatbot achieves 90% containment rate for common queries across 12 languages, particularly effective in India's diverse linguistic markets
Generative AI extends beyond just handling inquiries. It enables financial institutions to offer personalized financial advice based on proprietary data.
By training AI models on customers' financial goals, risk profiles, income levels, and spending habits, banks can provide highly customized budgeting and saving recommendations.
3. Fraud Detection on a Budget
Generative AI can be used to monitor transactions for anomalies (e.g., sudden large withdrawals) and alerts customers instantly. Study shows that community banks in the US adopted Generative AI slashed fraud losses by 45% using open-source AI tools.
We can use Generative AI Models trained on local spending patterns to reduce false positives for small businesses and gig workers.
AI models can adapt and learn from new data over time, continuously improving their accuracy and effectiveness of fraud prevention. This dynamic learning capability is crucial to counter - new techniques to evade detection .
- Anomaly detection: AI algorithms can instantly spot unusual patterns in transaction data.
- Customized risk assessment: Models trained on local data provide more accurate fraud detection for specific customer segments.
- Adaptive learning: AI systems continuously improve by learning from new transaction data and fraud patterns.
- Cost-effective implementation: Open-source tools make advanced fraud detection accessible to smaller financial institutions.
4. Compliance Automation
Generative AI can be used to extract data from loan documents and auto-fill regulatory templates (e.g., CRA reports), cutting manual work by 70%. Generative AI creates audit-ready summaries of customer interactions and transaction histories.
- Automated risk assessments: Generative AI models like GPT-4 can perform complex analyses to identify potential compliance issues, allowing for preemptive measures.
- Data analysis: AI tools can synthesize vast amounts of data to produce actionable insights for strategic compliance planning.
- Streamlined workflows: By automating routine tasks, generative AI empowers compliance officers to focus on more critical analytical work.
5. Dynamic Customer Notifications
AI analyzes transaction patterns to trigger real-time alerts (e.g., low-balance warnings, unusual spending spikes) via SMS or app notifications.
Here are some examples of How Generative AI generates alert outputs based on user specific data
Traditional Alert Messages:
1. "Low balance alert: $100"
2. "Unusual transaction detected"
3. "Payment due reminder"
Now Using Generative AI -we can craft dynamic, context-aware messages for the above by understanding customer profiles, transaction histories, and communication preferences.
1. "Your balance is approaching $100. Given your Netflix subscription renewal tomorrow and typical grocery spending, consider transferring funds from your savings."
2. "We noticed a $500 purchase at Electronics Store in Paris, while you're usually in New York. If this wasn't you, here's how to secure your account immediately..."
3. "Your mortgage payment is due in 3 days. Based on your current balance and expected deposits, you might want to adjust your discretionary spending this week. Here's a personalized plan..."
If you compare both you’ll get a clear idea how personalizing message alerts would be beneficial for your users.
Now if you look at the data Financial institutions implementing generative AI for notifications - report significant improvements in customer engagement and financial wellness.
Credit unions using advanced AI systems have seen a 30% reduction in overdraft fees through predictive notifications that not only warn about potential shortfalls but also generate personalized action plans 48 hours in advance.
6. AI-Driven Financial Health Tools
Generative AI can be used to create Budgeting Assistants which categorizes spending habits and generates personalized savings plans.
We’ve a partner who launched a "Financial Fitness" app that increased customer savings rates by 18% in six months using Generative AI.
Debt Management: AI simulates payoff scenarios (e.g., snowball vs. avalanche methods) based on income and liabilities.
7. Smart Document Search
Combining generative AI's contextual understanding with enterprise data for rapid information retrieval is how Smart Document search works
By using natural language processing (NLP) and machine learning (ML), these systems enable staff to ‘Query’ complex operational manuals, compliance guidelines, and customer records using conversational language eliminating keyword guesswork and manual searches. The LLM models summarizes the huge chunk of data and provides the answer the user is looking for.
Key Capabilities:
- Context-Aware Queries: Employees can ask questions like "Show me the 2025 AML policy updates for SME clients" and receive precise document excerpts with source citations.
- Multi-Format Processing: AI systems analyze PDFs, spreadsheets, emails, and scanned documents simultaneously through integrated OCR and deep learning.
- Dynamic Learning: The platform updates its knowledge base in real-time as new regulations, internal memos, or process documents are added.
8. Predictive Cash Flow Management
AI forecasts cash flow for small business clients, suggesting optimal invoice timing or loan refinancing. For a community bank we partnered with to integrate Gen AI.
We used AI to help local retailers reduce seasonal liquidity gaps by 40%.
9. Community-Specific Lending
Use alternative data (e.g., rent payments, social media activity) to serve underbanked populations. We’ve conducted a market study in which top Credit unions based on US boosted microloan approvals by 25% using AI to assess gig workers’ income stability.
How to Implement Generative AI in Banking and Finance Operations: Step-by-Step Guidelines
Generative AI is redefining banking and finance by automating intricate processes, improving decision accuracy, and minimizing operational risks.
To maximize its potential, institutions require a structured approach aligned with strategic priorities.
Here’s how to begin:
Step 1. Strategic Alignment & Use Case Scoping
Map institutional priorities (e.g., fraud detection, compliance reporting) to generative AI capabilities.
Partner with providers to identify gaps between existing workflows and AI-driven solutions.
For example, if you want to automate loan approval documents - specify requirements like data inputs, output formats, and compliance checks. Define success metrics (e.g., 40% faster report generation).
Key action:
Jointly draft a scope document with your IT partner outlining technical specifications, timelines, and accountability frameworks.
Step 2. Data Governance & Security Protocols
Audit data quality, accessibility, and sensitivity.
Generative AI requires clean, structured, and ethically sourced data. Share anonymized datasets with providers for model training while retaining control over sensitive information.
Ensure encryption and access controls comply with GDPR, CCPA, or regional regulations.
Key action:
Establish data-sharing agreements that limit provider access to only necessary datasets.
Step 3. Compliance & Risk Mitigation Frameworks
Align provider’s AI governance with financial regulations (e.g., FINMA guidelines, Basel III).
Require providers to demonstrate:
- Model explainability for audits (e.g., how credit decisions are made).
- Bias detection mechanisms in synthetic data generation.
- Real-time validation layers to prevent hallucinations in outputs.
Key action:
Integrate third-party audit clauses into contracts to validate model accuracy and fairness.
Step 4. Infrastructure & Technical Compatibility
Evaluate existing systems (e.g., core banking platforms) for AI integration.
Ensure the provider’s solution supports hybrid cloud setups for sensitive data isolation. Validate API compatibility for seamless data flow between legacy systems and AI tools.
Key action:
Conduct a compatibility workshop with IT teams, DevOps Engineers and the provider to identify integration bottlenecks.
Step 5. Pilot Testing & Validation
Run controlled Generative AI pilots for high-impact, low-risk workflows (e.g., automating customer service emails).
Measure performance against predefined KPIs (e.g., response accuracy, processing time). Use feedback to refine prompts, data inputs, or model architectures.
Key action:
Implement a “human-in-the-loop” phase where employees validate AI outputs before full automation.
Step 6. Ethical & Transparency Standards
Mandate ethical AI practices from providers.
Require clear labeling of AI-generated content (e.g., “This report was AI-drafted and reviewed by [Employee Name]”). Prohibit using customer data for model retraining without explicit consent.
Key action
Embed ethical AI clauses in contracts, including penalties for non-compliance.
Step 7. Continuous Monitoring & Adaptation
Establish oversight mechanisms for post-deployment.
Work with providers to schedule quarterly model updates using fresh transaction data or regulatory changes. Track performance degradation (e.g., rising false positives in fraud detection).
Key action:
Create a joint task force to review AI performance and prioritize updates.
Step 8. Contractual Safeguards & Exit Strategies
Negotiate service-level agreements (SLAs) that protect institutional interests.
Include clauses for:
- Data ownership and retrieval upon contract termination.
- Intellectual property rights for custom-built models.
- Penalties for breaches (e.g., data leaks, non-compliance).
Key action:
Why Partner with a Generative AI Service Provider for Your Financial Solutions?
Implementing generative AI in finance demands precision one misstep in compliance or data security can lead to costly setbacks.
At SayOne, we combine deep expertise in Generative AI development with a proven track record in financial outsourcing, ensuring integration of fraud detection systems, automated reporting, and personalized customer insights onto your existing workflow. Contact us today to get a quote!
Share This Article
Subscribe to Our Blog
We're committed to your privacy. SayOne uses the information you provide to us to contact you about our relevant content, products, and services. check out our privacy policy.