How to Build data-aware Gen AI applications with RAG (Use Cases & More)
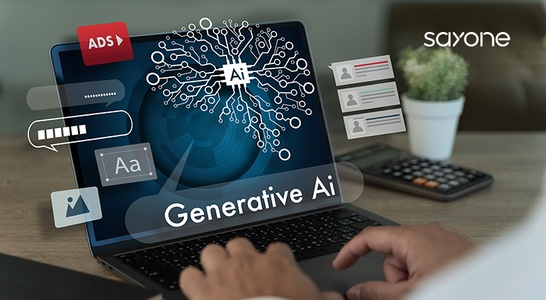
Share This Article
Table of Contents

Subscribe to Our Blog
We're committed to your privacy. SayOne uses the information you provide to us to contact you about our relevant content, products, and services. check out our privacy policy.
Customers expect hyper-personalized experiences, yet most businesses struggle to connect real-time data with AI’s decision-making power.
Most of the companies now use generative AI, but lack the talent to implement it effectively leaving gaps in accuracy and trust.
Entrepreneur, here’s your opportunity: the generative AI market is projected to grow 46% annually, with early adopters seeing $3.70 returned for every $1 invested.
But outdated approaches won’t cut it.
This is where retrieval-augmented generation (RAG) bridges the gap, turning your unstructured data, customer interactions, inventory logs, market trends into actionable, context-aware AI insights.
If you want AI chatbots that pull real-time stock updates, marketing campaigns that auto-adjust to regional buying patterns, or support tools that reduce misdiagnoses RAG can do it.
RAG makes this possible by grounding AI in your proprietary data, not generic models.
Let’s explore how data-aware Gen AI applications built with RAG are transforming retail and customer service and how you can lead the shift.
How Retrieval-Augmented Generation (RAG) Works
Retrieval-Augmented Generation (RAG) is an innovative AI framework that improves the capabilities of Large Language Models (LLMs) by grounding them in external knowledge sources. Instead of solely relying on pre-trained data, RAG models retrieve relevant information from a vector database like Qdrant to provide contextually rich and accurate responses.
The process begins with a user query. This query is then used to perform a similarity search within the vector database, identifying and retrieving the most relevant documents or passages. These retrieved pieces of information are then combined with the original prompt and fed into the LLM.
This augmented input allows the LLM to generate responses that are not only creative and coherent but also informed by up-to-date and specific knowledge. The results are AI outputs that are more trustworthy, factual, and tailored to the user's needs.
Benefits of Retrieval-Augmented Generation (RAG)
Retrieval-Augmented Generation (RAG) technology offers several benefits to an organization's generative AI efforts, making generative AI technology more broadly accessible and usable.
RAG enables generative AI systems to use external information sources to produce more accurate and context-aware responses, which is valuable for question-answering and content generation. By combining real-time data retrieval and the creative ability of text generation, RAG makes AI systems more accurate, contextually aware, and adaptable.
1. Cost-Effective Implementation
The computational and financial costs of retraining foundation models for organization or domain-specific information are too high. As an entrepreneur/business owner, you'll be glad to know that RAG is a more cost-effective approach to introducing new data to Large Language Models (LLMs).
Instead of retraining your entire model, RAG allows you to augment the LLM with your own data, which can be done with retrieval augmented generation (RAG).
This makes generative AI technology more broadly accessible and usable.
2. Access to Current Information
Staying relevant is a challenge, especially when original training data sources for an LLM may not be sufficient for your needs. You can use RAG to provide the latest research, statistics, or news to generative models.
Real-time data access RAG facilitates direct access to additional data resources so Generative AI solutions that use LLMs can remain up-to-date and current.
With Dynamic knowledge updates You can keep your external source up to date, so the retrieval system can search and provide the LLM with current and relevant information to generate responses. This eliminates the need to retrain a model on new data and update its parameters.
3. User Trust
Users always have mistrust on the information provided by your Generative AI system. With RAG, you can allow the LLM to present accurate information with source attribution.
The output can include citations or references to sources, and users can look up source documents themselves if they require further clarification or more detail.
This increases trust and confidence in your generative AI solution.
4. Real-Time Data Access for Accurate Insights
Customers expect answers based on the latest information, but traditional AI models often rely on outdated or generic data.
If Generative AI chatbot struggles to answer questions about recent market trends or policy changes. With RAG, you can ground responses in live data feeds, internal documents, or customer-specific databases.
For instance, financial institutions use RAG to pull real-time stock data or regulatory updates, ensuring every recommendation stays current.
Key capabilities:
- Dynamic knowledge integration: Automatically updates responses as your data sources evolve.
- Domain-specific relevance: Pulls insights from niche industry reports or proprietary datasets.
- Multi-source synthesis: Combines structured (spreadsheets) and unstructured (emails, PDFs) data seamlessly.
5. Cost-Effective AI Implementation
Training custom LLMs from scratch is expensive and time-consuming.
Why pour resources into retraining models when RAG lets you augment existing ones?
By connecting off-the-shelf LLMs to your curated data repositories, you sidestep the computational costs of full-scale retraining. A retail business adopting Generative AI, for example, could use RAG to align ChatGPT with its inventory system with no need to build a custom model.
6. Reducing Hallucinations & Building Trust
Generic AI often “makes up” answers, for the set of data they got.
Your customers won’t tolerate fabricated claims about product specs or pricing. RAG minimizes hallucinations by tethering responses to verified sources like CRM records or technical manuals.
Why this matters:
Users increasingly trust AI outputs more when sources are visible.
Support teams achieve faster resolution times with fact-checked AI suggestions.
Use Cases: Why do we Use Qdrants Vector Search Database?
1. AI-Powered Shopping Assistant
We created an AI-powered shopping assistant that offers personalized recommendations and instant customer support. Qdrant helped to index and retrieve product data and customer preferences in real-time, enabling the chatbot to deliver highly relevant suggestions.
For example, when a user asked, “Find summer dresses under $50 suitable for beach vacations,” the system combined semantic search with inventory filters to generate tailored suggestions.
Key Elements:
- Personalized Recommendations: SayOne used Qdrant to index customer behavior and product embeddings, enabling similarity searches for context-aware suggestions.
- 24/7 Query Resolution: Hybrid queries combined vectorized FAQs with live inventory data, reducing response latency. Qdrant's speed was a key component.
- Voice Commerce Integration: SayOne processed voice commands via vectorized intent recognition, improving accuracy in noisy environments. Qdrant helped to ensure voice commands were accurately translated into relevant searches.
2. Visual Search for Fashion Retailers
We developed a visual search solution that allows customers to find products by uploading images. For a client’s app, Qdrant matched user-uploaded images with similar products. For example, a customer photographed an outfit and uploaded it to our search engine, and the system identified near-identical items from a vast catalog using multimodal embeddings.
Key Features:
- Image-to-Product Matching: SayOne stored embeddings of product images, enabling fast similarity searches, with the help of Qdrant.
- Trend Analysis: Clustered frequently searched visual patterns to predict emerging styles, reducing deadstock. Qdrant helped to quickly identify visual trends from a large number of searches.
3. Dynamic Pricing Engine
SayOne built a dynamic pricing engine that adjusts prices in real-time by analyzing competitor data, demand trends, and margins. With the support of Qdrant’s payload-aware vectors, this system was able to quickly analyze and respond to market changes. For example, during flash sales, the system auto-discounted overstocked items while premium products saw price hikes.
Impact:
- Competitor Benchmarking: SayOne scraped pricing data stored as vectors, enabling instant similarity comparisons across retailers, using Qdrant to accelerate this process.
- Demand Forecasting: Hybrid queries combined sales history and weather data embeddings to predict regional demand spikes.
- Margin Optimization: AI-generated pricing strategies improved gross margins, allowing SayOne to achieve better results for their clients.
By integrating Qdrant into its generative AI solutions, We have achieved faster customer query resolution, higher average order values, and reduced operational costs for our retail clients.
Conclusion
Retrieval-Augmented Generation (RAG) is a powerful framework for developing data-aware GenAI applications, offering numerous benefits.
By grounding language models in external knowledge sources, RAG enhances accuracy, reduces hallucination, and enables real-time adaptability. The integration of vector search databases like Qdrant further optimizes RAG pipelines, allowing for efficient retrieval of relevant information.
As the field of GenAI continues to evolve, RAG will undoubtedly play a crucial role in unlocking the full potential of these models and delivering more reliable and contextually relevant solutions across various industries.
Share This Article
Subscribe to Our Blog
We're committed to your privacy. SayOne uses the information you provide to us to contact you about our relevant content, products, and services. check out our privacy policy.