The role of a Business Analyst in the age of AI
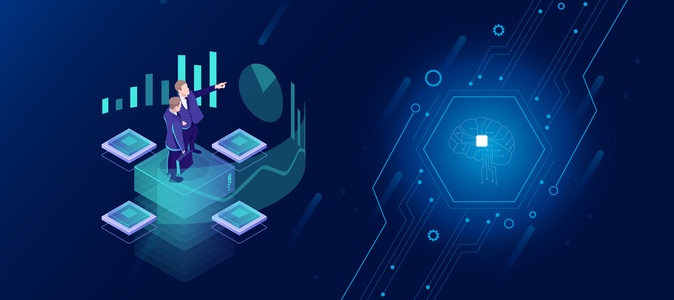
Share This Article
Table of Contents

Subscribe to Our Blog
We're committed to your privacy. SayOne uses the information you provide to us to contact you about our relevant content, products, and services. check out our privacy policy.
Are you a Business Analyst who worries how to handle a project that integrates Artificial Intelligence and Machine Learning or do you think whether a Business analyst has any role at all in that kind of projects?
Of course Yes! Business Analysis has got the same impact on an AI/ML project like that on the other projects. But being a business analyst whether you can make the same impact on these projects depends purely on your skills and your adaptability.
To put AI ML in simple words, you're giving the computer the data and tools to study a problem and solve it without human intervention. You're giving the computer the ability to introspect what it did so it can adapt, evolve, and learn. Thus the 9 key steps in the ai ml process are listed below.
-
Gathering Data
-
Data Preparation
-
Choosing a model
-
Training
-
Evaluation
-
Parameter tuning
-
Prediction
-
Test
-
Deploy
The first 2 steps are the basis of the AI ML. The quality and quantity of the data determine how good, useful and accurate the prediction will be. Make a mistake here, no matter how good the development strategy is, and how good the algorithm is, the product is not going to be fruitful as expected. And this is where being a Business Analyst you can play an important role. The lion’s share of your role will be in these 2 stages.
Before narrating how to play the business analyst role in AI ML projects, some of the challenges that are faced by many of the organizations/developers while adopting AI/ML.
-
Gathering the exact requirements of the project.
-
Ambiguous feature list.
-
Unclear product roadmap.
-
Finding KPIs to generate insights relevant for decision making.
-
Lack of knowledgeable resources
-
Choosing the right tech stack.
When we analyze these challenges, what we can infer is that the impediments were not choosing an algorithm or training the system with the data, but it was with the first milestone. Over the course of time, the team may get hindered with some significant technical challenges, but the expertise and online guidance will help the team get a solution to it. But a mistake on the first milestone will lead to a faulty product and waste of time. That's how important is the role of an efficient BA.
Let’s start breaking down how a BA can make up to be of help in these challenges.
1. Know what is AI/ML
A business analyst need not be tech-savvy, but like in any other projects, you need to have an understanding of AI/ML. One of the existing challenges is the gap between skilled data scientists and business leaders who know how to drive the technology, and without no doubt, a knowledgeable BA can fill this gap.
One liner requirement for an AI ML project always sounds like predicting the value/price/outcome of something in the future. This will sound a little unfamiliar, but when we know how AI/ML works, at least we will know how to approach the requirement elicitation.
2. Know the Domain
Forrester recently reported that almost two-thirds of enterprise technology decision-makers have either implemented/ implementing, or are expanding their use of AI. AI/ML is having its applicability across all industries and domains. As knowing the domain is crucial for the efficiency of business analysis in any other projects in these projects also the domain knowledge has got the impact. How thorough you know the domain that efficiently you can be in contributing to the success.
3. Identify the right data repositories
The data might be scattered throughout the organization, or sometimes the data that we need might not be readily available. Data may not have been collected or labelled yet or may not be readily available for machine learning model development because of technological, budgetary, privacy, or security concerns. This varies for projects, and in some business contexts, the stakeholders will have to see how reliable the system is before investing their fund and time in collecting, labelling and moving the data. There are many data sets you can find online for any use cases. But the team requires to know what is the availability of data which will also help them to plan the model and timeline.
4. Formulate a strategic approach
The two major challenges from the above-mentioned list are ambiguous feature list and finding KPIs to generate insights relevant for decision making. The analysis reveals that these challenges exist due to the absence of a strategic approach/plan. Below are the stages of the plan.
-
Align Goals to business objectives: Get to know what are the organization’s objectives behind the project and what defines success. Then align the technical/project activities with those objectives
-
Identify KPIs: Key Performance Indicators separate meaningful information from an abundance of data. To know if we are successful in meeting the objectives there’s a KPI to prove it. Identify those indicators.
-
Track ROI: Track the results to validate how right you were about the KPIs and to know how accurate the system is.
-
Conduct Feedback gathering sessions.
An AI/ML product requires continuous improvement based on the feedback. There require many iterations by testing it with the test data. The Business Analyst is responsible to find the right stakeholders to include in the feedback session. Their feedback drives the project in the right discussion. Gather the feedback, document it and pass it to the team to look out in the next milestone.
when the business analysis is done the right way the above-discussed challenges won’t be a big challenge at all to deal with. It is evident that with any technology the business analysis and the business analyst need to evolve to adapt the nature and characteristics. Being said that the AI/ML also requires the business analyst to take the best of their skills, apply them in the modern context.
No matter what the project or technology is, business analysis has got a role to play. And as it comes in the initial phase, any imperfections in the requirement will lead to frequent rework.
Share This Article
Subscribe to Our Blog
We're committed to your privacy. SayOne uses the information you provide to us to contact you about our relevant content, products, and services. check out our privacy policy.